Hans Hao-Hsun Hsu
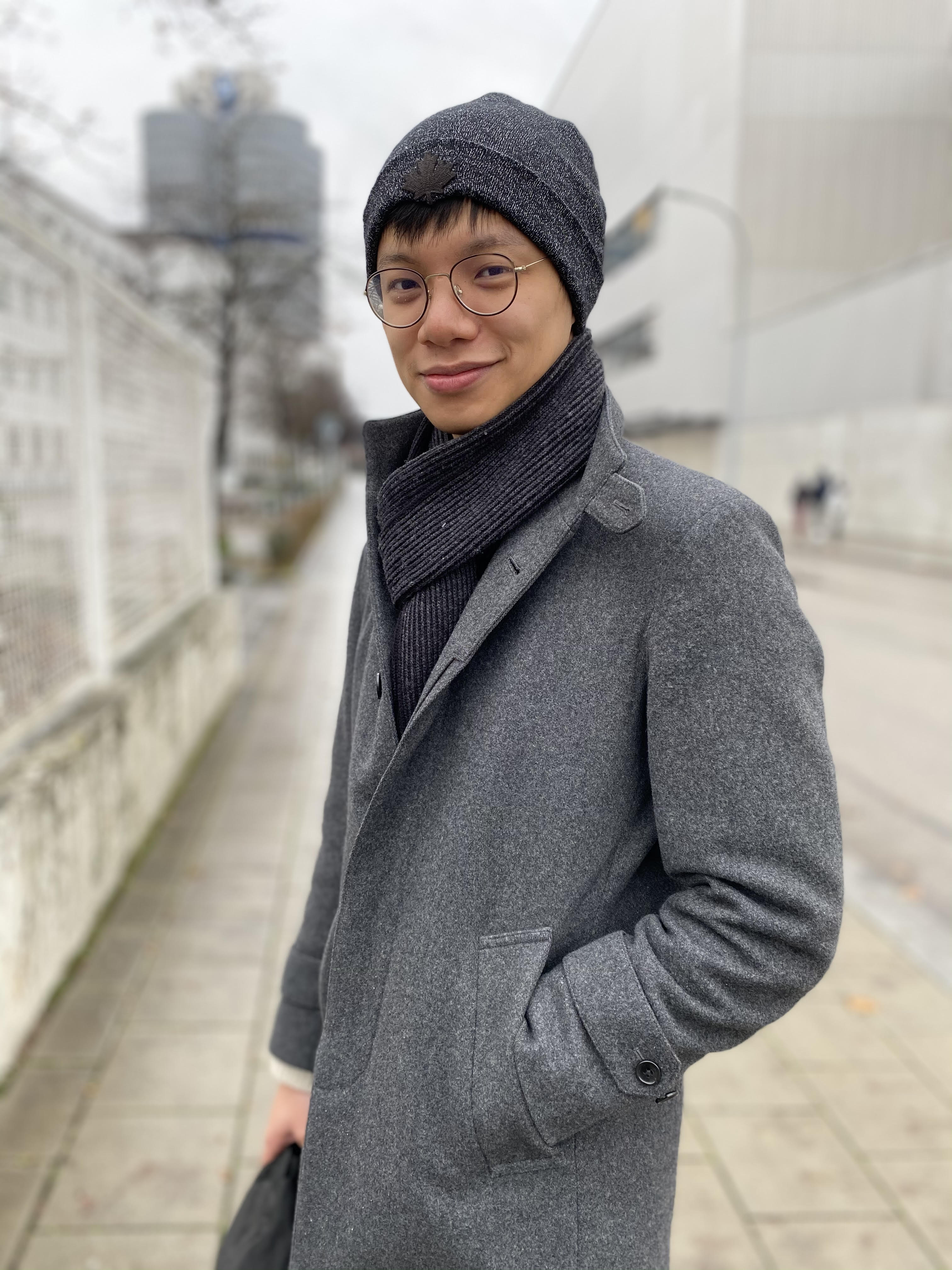
Hi, my name is Hans Hao-Hsun Hsu. I am currently a research intern at Georgia Tech where I work with Pan Li on domain adaptation in graph machine learning. I am also looking for PhD positions starting in Fall 2025.
My current research interests lie in (1) geometric deep learning and its application to scientific discovery/engineering (2) trustworthy AI on graphs (reliability, robustness and explainability). My long term goal is to integrate these two areas, developing machines that are both reliable and applicable in scientific scenarios (usually characterized by data scarcity).
Previously, I was a machine learning researcher at Celeris Therapeutics, an Austria- and UK-based startup, working on PROTACs-related drug discovery projects. I obtained my M.S. degree (with high distinction) from the Technical University of Munich advised by Daniel Cremers, where I worked on uncertainty estimation in geometric deep learning. I also hold a B.S. degree from National Taiwan University.
Feel free to reach out to me for any collaboration or research opportunities.
selected publications
-
- Automated Antenna Testing Using Encoder-Decoder-based Anomaly DetectionIn International Conference on Machine Learning and Applications (ICMLA) , 2021
other research projects
- GraphPROTACs: Out Of Distribution DC50 PredictionCeleris Therapeutics, 3.2023 - 1.2024
Curated a PROTAC dataset and proposed GraphPROTACs, achieving state-of-the-art generalizability (AUROC increased from 55% to 75%) and enhanced model interpretability, with further improvements from training on synthetic data.